The Power of Hyperspectral Satellite Imaging: Applications and Future Directions
This article delves into the fascinating world of hyperspectral satellite image processing, discussing its applications in agriculture, environmental monitoring, mineral exploration, and defense. We explore the challenges and limitations of this technology and its potential future directions, providing insights into how hyperspectral imaging can help us better understand and manage our planet's resources.
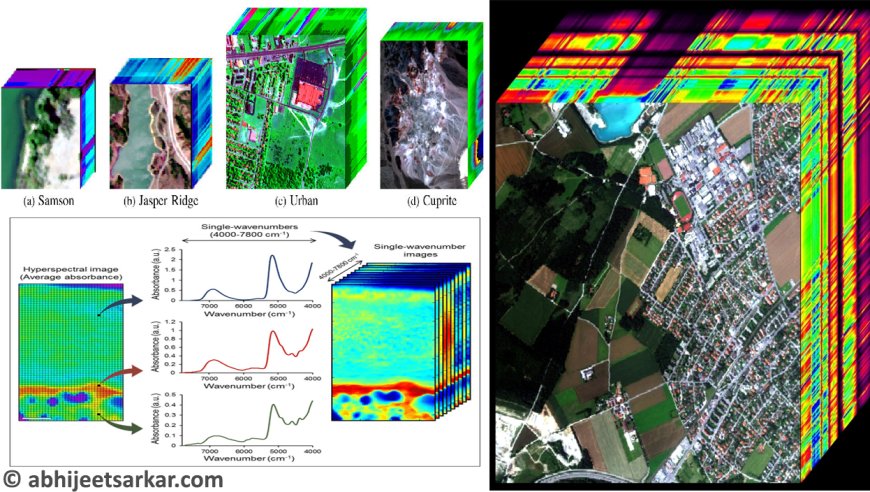
Hyperspectral remote sensing technology has revolutionized the field of earth observation by providing detailed spectral information about the earth's surface. Hyperspectral sensors collect data in narrow, contiguous spectral bands that cover the entire electromagnetic spectrum. The number of bands in a hyperspectral image can range from tens to hundreds, allowing for detailed characterization of the spectral properties of materials and phenomena on the earth's surface.
Hyperspectral bands provide a wealth of information about the reflectance properties of materials and phenomena on the earth's surface. By analyzing the spectral properties of hyperspectral bands, it is possible to identify and map different materials and vegetation types, monitor changes in land cover and land use, and study the physical and chemical properties of soils and water bodies.
In this article, we will explore the basics of hyperspectral bands, including how they are collected, processed, and analyzed. We will also discuss some of the applications of hyperspectral bands, including mineral mapping, vegetation analysis, and land cover classification.
Hyperspectral Bands:
Hyperspectral bands are contiguous spectral bands that cover the entire electromagnetic spectrum. These bands are typically very narrow, ranging from a few nanometers to a few tens of nanometers in width. The number of hyperspectral bands in a dataset can range from tens to hundreds, depending on the specific sensor used and the resolution of the data.
The spectral resolution of hyperspectral bands is one of their defining characteristics. Spectral resolution refers to the ability of a sensor to distinguish between different wavelengths of light. Higher spectral resolution allows for more detailed characterization of the spectral properties of materials and phenomena on the earth's surface.
Hyperspectral sensors use a variety of techniques to achieve high spectral resolution, including prism-based spectrometers, diffraction gratings, and interference filters. These sensors collect data in narrow, contiguous spectral bands that cover the entire electromagnetic spectrum, from the visible to the thermal infrared.
Hyperspectral bands are typically represented as a spectrum, which shows the reflectance properties of a material or phenomenon across the entire electromagnetic spectrum. The shape of the spectrum can provide information about the chemical and physical properties of the material, including its absorption and scattering properties.
Hyperspectral bands are often processed and analyzed using a variety of techniques, including principal component analysis (PCA), spectral angle mapping (SAM), and linear unmixing. These techniques allow for the extraction of meaningful information from hyperspectral bands, including the identification and mapping of different materials and phenomena on the earth's surface.
Applications of Hyperspectral Bands:
Hyperspectral bands have a wide range of applications in earth observation and remote sensing. Some of the most common applications of hyperspectral bands include:
-
Mineral Mapping: Hyperspectral bands can be used to map the distribution and composition of minerals on the earth's surface. Different minerals have characteristic spectral properties that can be identified and mapped using hyperspectral data. Mineral mapping is important for a variety of applications, including resource exploration, environmental monitoring, and land management.
-
Vegetation Analysis: Hyperspectral bands can be used to monitor the health and productivity of vegetation on the earth's surface. Different vegetation types have characteristic spectral properties that can be used to identify and map different types of vegetation. Vegetation analysis is important for a variety of applications, including agriculture, forestry, and environmental monitoring.
-
Land Cover Classification: Hyperspectral bands can be used to classify different land cover types on the earth's surface. Different land cover types have characteristic spectral properties that can be used to identify and map different types of land cover. Land cover classification is important for a variety of applications, including land management, urban planning, and environmental monitoring.
-
Atmospheric Correction: Hyperspectral bands can be used to correct for atmospheric effects in remote sensing data. The atmosphere can affect the spectral properties of materials on the earth's surface, leading to errors in remote sensing data. Hyperspectral bands can be used to correct for these effects, allowing for more accurate analysis of remote sensing data.
-
Water Quality Monitoring: Hyperspectral bands can be used to monitor the quality of water bodies, including lakes, rivers, and oceans. Different water quality parameters have characteristic spectral properties that can be identified and mapped using hyperspectral data. Water quality monitoring is important for a variety of applications, including water resource management, environmental monitoring, and public health.
-
Geology: Hyperspectral bands can be used to study the geology of the earth's surface, including the identification and mapping of different rock types, geological structures, and mineral resources. This information is important for a variety of applications, including resource exploration, environmental monitoring, and land management.
Challenges of Hyperspectral Bands:
While hyperspectral bands provide a wealth of information about the earth's surface, there are also several challenges associated with their use.
-
Data Volume: Hyperspectral datasets can be very large, with hundreds or thousands of spectral bands. This can make it difficult to process and analyze the data, particularly for applications that require real-time or near-real-time analysis.
-
Spectral Complexity: Hyperspectral bands can be very complex, with many overlapping spectral features. This can make it difficult to identify and map different materials and phenomena on the earth's surface.
-
Spectral Calibration: Hyperspectral sensors require careful calibration to ensure accurate and consistent data. Calibration is particularly important for applications that require accurate spectral measurements, such as mineral mapping and vegetation analysis.
-
Atmospheric Effects: The atmosphere can affect the spectral properties of materials on the earth's surface, leading to errors in hyperspectral data. Atmospheric correction is often required to correct for these effects.
-
Cost: Hyperspectral sensors can be expensive, making it difficult to acquire high-quality data for some applications.
Hyperspectral Band Combinations
Hyperspectral data typically consists of hundreds of spectral bands, making it difficult to interpret the data and extract meaningful information. One approach to address this challenge is to combine or subset the spectral bands to enhance specific features of interest. Here are some commonly used hyperspectral band combinations:
-
False Color Composite (FCC): This combination involves assigning different wavelengths to the red, green, and blue color channels to create an image that highlights specific features. For example, in a vegetation study, NIR (Near Infrared) can be assigned to the red channel, green to the green channel, and blue to the blue channel, creating an FCC that enhances vegetation health.
-
Principal Component Analysis (PCA): PCA is a statistical method that identifies the most significant spectral features in a hyperspectral dataset and creates new bands by linearly combining the original bands. The resulting bands, called principal components, can be used to highlight specific features, such as land cover or mineralogy.
-
Ratio and Index Combinations: Ratios and indices are commonly used to quantify specific features in a hyperspectral dataset. For example, the Normalized Difference Vegetation Index (NDVI) is commonly used to measure vegetation health by calculating the ratio of NIR to red reflectance. Other indices such as the Soil Adjusted Vegetation Index (SAVI) and the Chlorophyll Absorption Ratio Index (CARI) can be used to quantify vegetation stress and chlorophyll content, respectively.
-
Spectral Angle Mapper (SAM): SAM is a classification technique that compares the spectral signature of a pixel to a known spectral signature. This comparison is performed by calculating the spectral angle between the two signatures. SAM can be used to identify specific materials or land cover types based on their unique spectral signatures.
-
Minimum Noise Fraction (MNF): MNF is a method that reduces noise in a hyperspectral dataset by transforming the data into a lower-dimensional space. This transformation creates new bands that capture the most significant spectral information while minimizing noise. MNF can be used to enhance specific features or reduce noise in a hyperspectral dataset.
-
Tasseled Cap Transform (TCT): TCT is a linear combination of the hyperspectral bands that are designed to represent specific physical properties of the observed scene. It is commonly used in remote sensing applications to classify land cover and monitor changes over time. TCT provides six coefficients that can be used to generate three composite images that highlight soil, vegetation, and water content.
-
Band Ratios for Mineral Mapping: Certain minerals exhibit unique spectral features that can be used to identify them in a hyperspectral dataset. For example, iron oxides can be identified by calculating the ratio of reflectance at 0.43 and 0.86 micrometers, while kaolinite can be identified by calculating the ratio of reflectance at 2.2 and 2.35 micrometers.
-
Continuum Removal: Continuum removal is a technique that removes the spectral continuum from the hyperspectral data to enhance absorption features. This method is particularly useful for identifying specific chemical compounds in the data. Continuum removal can be used to detect and map hydrocarbons, minerals, and vegetation pigments.
-
Normalized Difference Water Index (NDWI): NDWI is a ratio-based index that is commonly used to map surface water. It is calculated by subtracting the NIR band from the SWIR (Shortwave Infrared) band and dividing the result by their sum. NDWI can be used to detect and map changes in surface water over time.
-
Red-Edge Inflection Point (REIP): REIP is the wavelength at which the slope of the reflectance curve changes rapidly in the red-edge region of the spectrum. This feature is particularly useful for vegetation studies as it can be used to estimate chlorophyll content, leaf area index, and biomass. REIP can also be used to distinguish between different types of vegetation and identify stress-induced changes in plant physiology.
The number of hyperspectral band combinations that can be created from a dataset with hundreds of spectral bands is virtually unlimited. However, some commonly used combinations include:
- False Color Composite (FCC) - 3 bands
- Principal Component Analysis (PCA) - a reduced set of bands, typically 3-10
- Ratio and Index Combinations - 1 or 2 bands
- Spectral Angle Mapper (SAM) - 1 or 2 bands
- Minimum Noise Fraction (MNF) - a reduced set of bands, typically 3-10
- Tasseled Cap Transform (TCT) - 3 bands
- Band Ratios for Mineral Mapping - 2 bands
- Continuum Removal - a reduced set of bands, typically 3-10
- Normalized Difference Water Index (NDWI) - 2 bands
- Red-Edge Inflection Point (REIP) - 1 band
- Continuum-Removed Spectra (CRS) - a reduced set of bands, typically 3-10
- Wavelet Transform - a reduced set of bands, typically 3-10
- Spectral Mixture Analysis (SMA) - a set of 2-3 endmember spectra
- Feature-oriented Principal Component Analysis (FPCA) - a set of 2-3 basis spectra
- Maximum Autocorrelation Factor (MAF) - a reduced set of bands, typically 3-10
- Independent Component Analysis (ICA) - a reduced set of bands, typically 3-10
- Non-negative Matrix Factorization (NMF) - a reduced set of bands, typically 3-10
These techniques are often used in specific applications such as mineral mapping, vegetation analysis, and land cover classification. The number of bands used in each technique depends on the specific goals and requirements of the analysis.
Overall, the number of possible hyperspectral band combinations is vast and depends on the particular application and data being analyzed. Therefore, it's important to carefully consider the goals and requirements of the analysis when selecting an appropriate band combination or processing technique.
The selection of hyperspectral band combinations depends on the specific goals and requirements of the analysis. Here are some factors to consider when selecting hyperspectral band combinations:
-
Spectral Features: Consider the spectral features of the materials or phenomena of interest in the scene. Identify the wavelengths where these features occur and select the bands that capture those wavelengths.
-
Noise and Interference: Noise and interference can affect the quality of hyperspectral data. Selecting bands that are less affected by noise and interference can improve the accuracy of the analysis.
-
Band Correlation: Hyperspectral bands are often highly correlated, meaning that they contain similar information. Selecting bands that are less correlated can reduce redundancy and improve the efficiency of the analysis.
-
Spatial Resolution: Hyperspectral datasets can have different spatial resolutions. Selecting bands that have the same spatial resolution can ensure that the analysis is consistent across the entire scene.
-
Application-Specific Requirements: The selection of hyperspectral bands also depends on the specific requirements of the application. For example, some applications may require a small number of bands for efficient processing, while others may require a larger number of bands for improved accuracy.
Hyperspectral Satellite Image Processing
Hyperspectral satellite image processing is a powerful technique that allows scientists to extract detailed information from satellite images. Unlike traditional satellite imagery, which captures data in a few spectral bands, hyperspectral imaging captures data in hundreds of contiguous spectral bands, providing a wealth of information about the composition and properties of the earth's surface. In this blog post, we will explore the basics of hyperspectral satellite image processing and its applications in various fields.
What is Hyperspectral Imaging?
Hyperspectral imaging is a technique that captures the spectral signature of every pixel in an image. Spectral signatures are the unique patterns of energy that objects emit or reflect at different wavelengths of the electromagnetic spectrum. These patterns can reveal a wealth of information about the object's composition, physical properties, and environment.
Hyperspectral imaging sensors capture data in contiguous spectral bands, ranging from visible light to near-infrared and thermal radiation. Each band represents a narrow range of wavelengths, typically 5-10 nanometers wide. By analyzing the spectral signature of each pixel in an image, scientists can identify the materials and substances present on the earth's surface.
Hyperspectral Satellite Image Processing
Hyperspectral satellite image processing involves a series of complex algorithms and techniques to extract information from the raw spectral data. The goal is to identify and classify materials and substances on the earth's surface accurately.
The first step in hyperspectral satellite image processing is to preprocess the data. This involves correcting for atmospheric distortion, removing noise, and calibrating the data to account for the sensor's characteristics. Once the data is preprocessed, the next step is to perform spectral unmixing. Spectral unmixing is a process that separates the spectral signature of each pixel into its constituent parts, typically representing different materials or substances.
The spectral unmixing algorithm uses a library of spectral signatures to identify the materials present in the image. The library may contain spectral signatures for various vegetation types, minerals, water, and other substances. By comparing the spectral signature of each pixel to the library, the algorithm can identify the materials present in each pixel.
Once the materials are identified, the next step is to classify them into different categories. For example, vegetation may be classified into different types based on its health, species, or density. Similarly, minerals may be classified based on their composition or concentration. The classification algorithm uses statistical techniques and machine learning algorithms to assign each pixel to a specific category.
Applications of Hyperspectral Satellite Image Processing
Hyperspectral satellite image processing has a wide range of applications in various fields, including agriculture, environmental monitoring, mineral exploration, and defense.
Agriculture: Hyperspectral imaging can be used to monitor crop health and identify nutrient deficiencies or pest infestations. By analyzing the spectral signature of crops, scientists can detect subtle changes in plant physiology that may indicate stress or disease. This information can be used to optimize crop management practices and improve yields.
Environmental Monitoring: Hyperspectral imaging can be used to monitor changes in land cover, identify pollution sources, and monitor water quality. For example, the spectral signature of water bodies can reveal the presence of pollutants or algae blooms. Hyperspectral imaging can also be used to map wetlands, forests, and other ecosystems, providing valuable information for conservation efforts.
Mineral Exploration: Hyperspectral imaging is widely used in mineral exploration to identify and map mineral deposits. The spectral signature of minerals can reveal their composition and concentration, allowing geologists to locate and map mineral deposits. This information is critical for mineral exploration companies to make informed decisions about where to drill and invest resources.
Defense: Hyperspectral imaging can be used for a wide range of defense applications, including target detection, surveillance, and intelligence gathering. The spectral signature of materials can reveal their properties, such as reflectivity,transparency, and chemical composition. This information can be used to detect and identify targets, such as vehicles or buildings, even in challenging environments or camouflage.
Challenges and Future Directions
While hyperspectral satellite image processing has many applications, there are also some challenges and limitations to the technology. One of the main challenges is processing the large amounts of data generated by hyperspectral sensors. The sheer volume of data can be overwhelming, requiring specialized software and hardware to handle it efficiently.
Another challenge is the need for accurate spectral libraries. The accuracy of the spectral unmixing algorithm depends on the quality and completeness of the library used to identify materials. Developing and maintaining accurate spectral libraries is an ongoing challenge for scientists working in the field.
Despite these challenges, hyperspectral satellite image processing is a rapidly evolving field, with many exciting opportunities for future research and development. Some areas of active research include developing more efficient algorithms for processing hyperspectral data, improving spectral libraries, and integrating hyperspectral data with other remote sensing technologies, such as LiDAR or radar.
As hyperspectral satellite image processing continues to evolve, it is likely to have an even greater impact on a wide range of industries and scientific fields. For example, in agriculture, hyperspectral imaging could be used to monitor crop health in real-time, allowing farmers to make informed decisions about irrigation, fertilization, and pest control. In environmental monitoring, hyperspectral imaging could be used to detect and monitor harmful algal blooms, pollution sources, and changes in land cover due to climate change.
In mineral exploration, hyperspectral imaging could be used to map the distribution and concentration of minerals in three dimensions, allowing mining companies to make more informed decisions about where to drill and invest resources. In defense, hyperspectral imaging could be used for a wide range of applications, such as detecting and identifying improvised explosive devices, monitoring border crossings, and tracking the movement of military vehicles and personnel.
Conclusion
In conclusion, hyperspectral satellite image processing is a powerful and rapidly evolving technology that provides valuable insights into the composition and properties of the earth's surface. While the technology has some challenges and limitations, it is likely to have an even greater impact on a wide range of industries and scientific fields in the coming years. As the technology continues to evolve, we can expect to see new applications and opportunities for research and development, making hyperspectral imaging an essential tool for understanding and managing our planet's resources.
Hyperspectral satellite image processing is a powerful technique that provides a wealth of information about the earth's surface. By analyzing the spectral signature of each pixel in an image, scientists can identify and classify materials and substances, providing valuable insights into agriculture, environmental monitoring, mineral exploration, and defense. While the technology has some challenges and limitations, it is a rapidly evolving field with many exciting opportunities for future research and development.
What's Your Reaction?
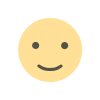
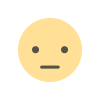
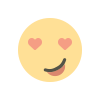
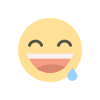
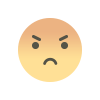
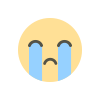
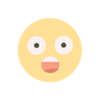